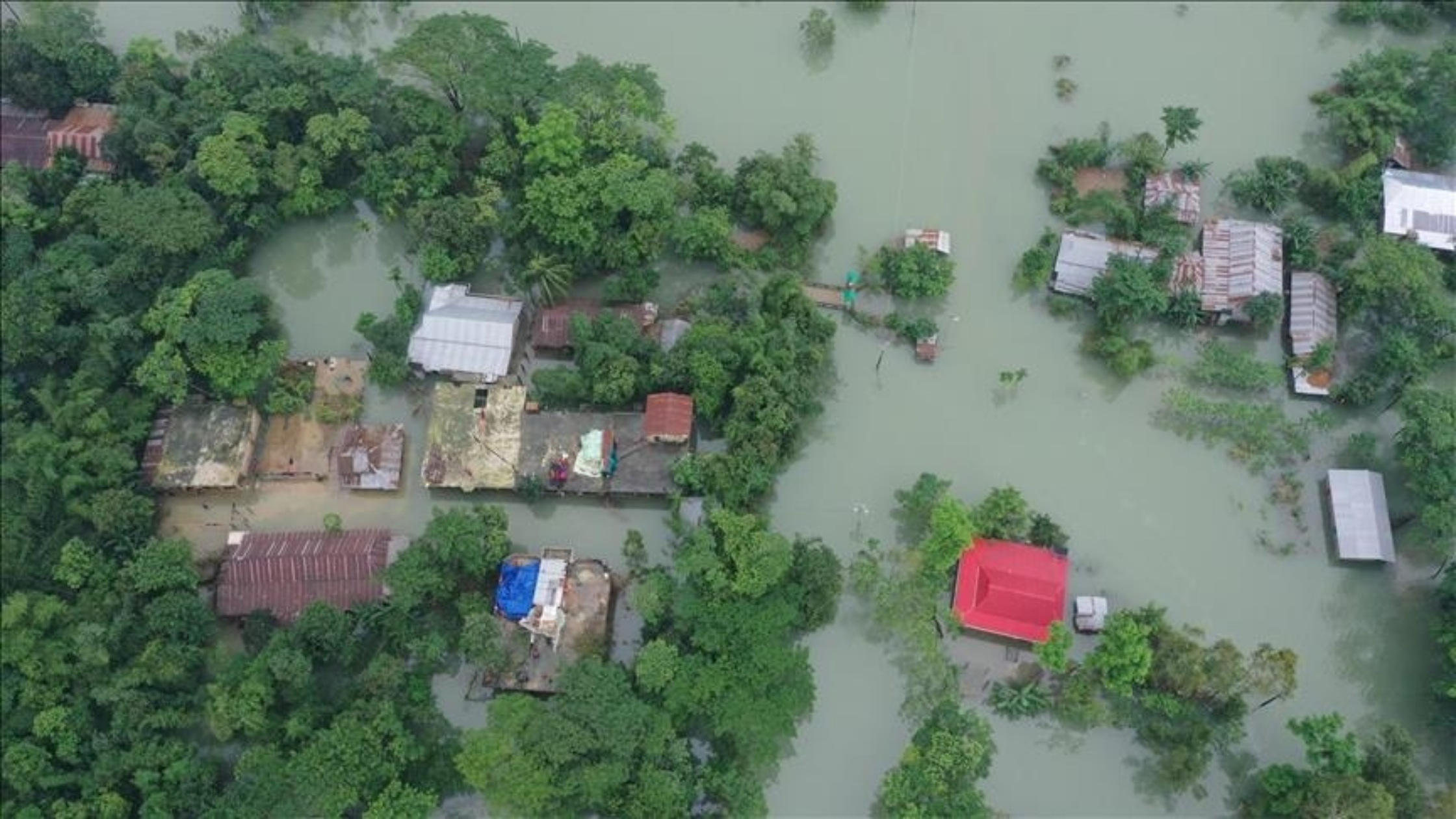
Bangladesh is one of the most vulnerable countries in the world to a changing climate
The Sylhet region of Northeast Bangladesh continues to recover from a series of unprecedented flooding events that occurred in the months of May and June of this year. Atiqul Haque, the Director General of Bangladesh’s Department of Disaster Management, reported that the flooding was the worst in 122 years leaving wide areas of Sylhet submerged and disconnected from the rest of the country. Humanitarian agencies in the area have reported that 4.3 million people have been impacted directly by the flooding, leaving an estimated 384,000 people displaced. Months after the initial reporting, flooding in some areas has receded, leaving hundreds of thousands of Bangladeshis shelter less.
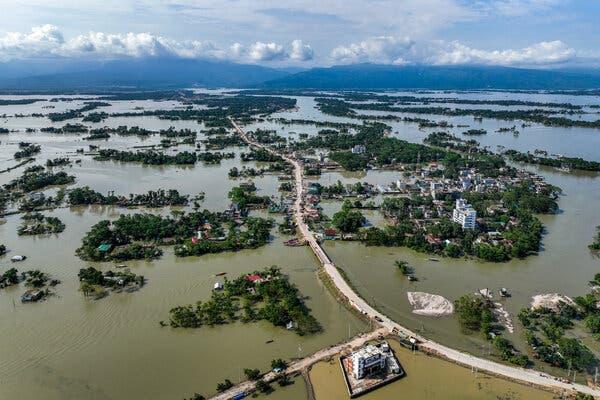
These floods may well be only a warning sign of what is to come in the years ahead. Bangladesh is one of the world's most vulnerable countries to climate change, responsible for driving sea level rise, extreme flooding events, and intensifying tropical storms. Already more than 10 million Bangladeshis have become “climate migrants” according to Bangladesh.
State Minister for Foreign Affairs. A USAID government report in 2018 found that 90 million Bangladeshis, 56% of the total population, live in high-risk climate exposure areas. The same report estimates that one in every seven people in Bangladesh will be displaced by climate change by the year 2050.
RAMP (Replicable AI for Microplanning) is changing the way we respond to disasters
Advances in Machine Learning (ML) and Artificial Intelligence (AI) are opening the door for innovative solutions that aim -to tackle some of the most pressing environmental and social problems of our time. For context, artificially intelligent machine learning models are algorithms that have been taught by humans how to recognize patterns in data. We interact with these technologies in our everyday lives more than we know (e.g., when we use a map on our phone when something we type is autocorrected, when we use our phones to identify a song). RAMP is a machine learning technology that has been taught to accurately detect and capture building footprints from satellite imagery taken from space. The RAMP model and its building footprint data products can be used for a variety of applications such as disaster planning, population estimation, and health microplanning. Disaster response, another one of RAMP’s main applications, can provide invaluable in the context of an event like the flooding that took place in Sylhet this spring.
The first thing that needs to take place after a disaster occurs is understanding the impact it has had on human lives. Rapid response teams begin gathering as much data as possible from existing national and sub-national records to determine the population distribution in the affected areas. Understanding how many people are affected and where they are located is the foundational and most critical stage in a disaster response effort. It is also often the most difficult. In many parts of the world, data on these critical insights have varying levels of accuracy and are often incomplete or do not exist together. In addition, there is no immediate way to update the data aside from doing so by hand, which is an incredibly time-consuming task.
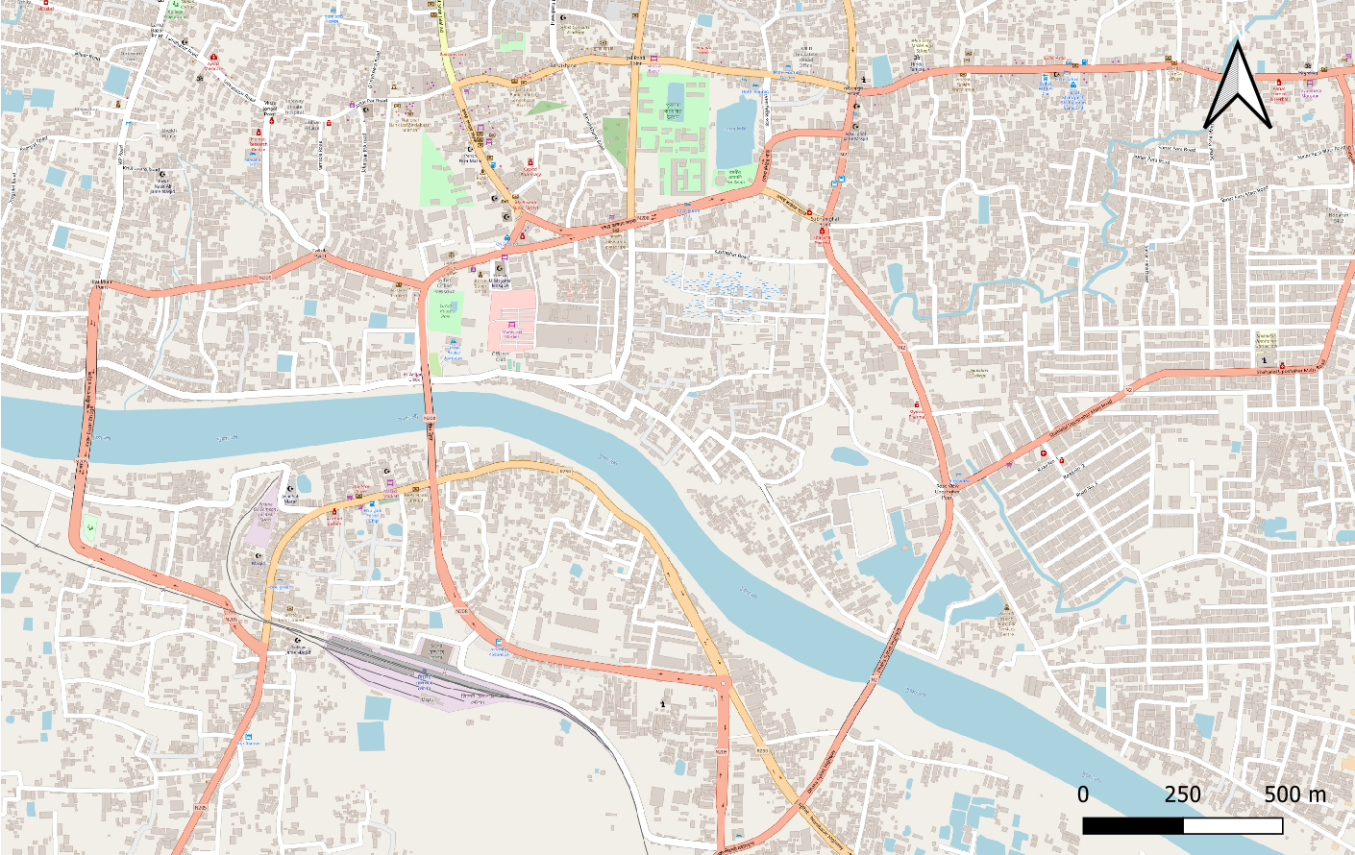
RAMP changes the playing field by greatly reducing the time needed to create an updated map of where buildings existed prior to the flood event. The rapid response team would only need to feed the machine learning model a small set of training data to localize the model so it can accurately extract building footprints against the most recent satellite imagery. RAMP comes with its own hurdles for use of course, but the infrastructure can be set up and in place before a disaster even strikes. The overall reduction in time associated with data creation can then be re-purposed towards planning the response with a more accurate and recent map. All of this enables more effective identification of vulnerable populations, planning navigation routes, and the distribution of life-saving medical supplies.
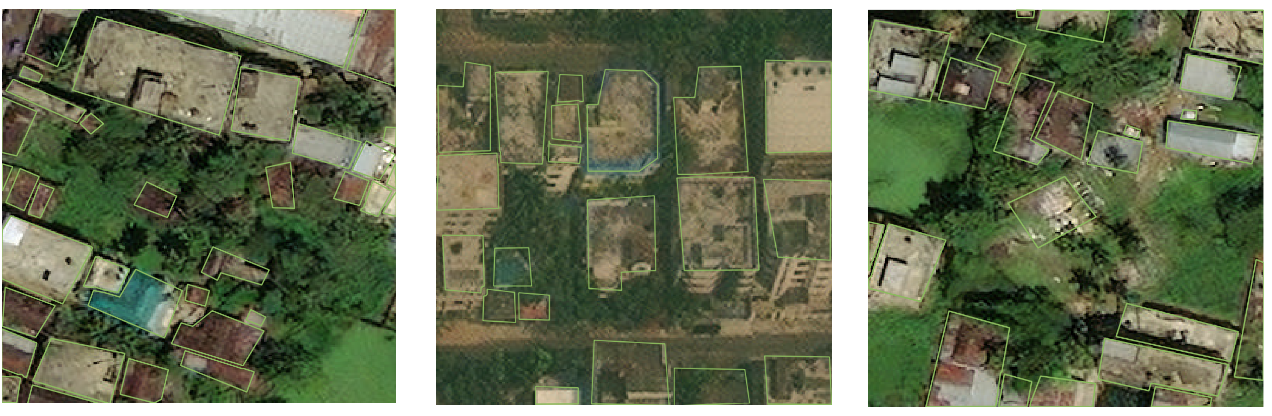
RAMP was developed to democratize AI and ML for use in humanitarian applications by lowering the bar for entry into building extraction using satellite imagery
Other projects in the past led by large commercial companies like Facebook and Google have developed similar models to RAMP. The projects have released building footprint data across huge geographic areas providing invaluable insights to humanitarian organizations such as the WHO and UNICEF, but these projects have their limitations. The models and documentation on how the data was created are not publicly released, meaning these tech giants essentially hold the keys to where and when the data is produced. Other platforms like Open Street Map serve as a great open data platform, but buildings are generated manually through crowdsourcing, and it is not a pathway to large-scale data creation. RAMP addresses these limitations, building on these past efforts and taking things one step further by making every aspect of the technology openly accessible, replicable, and extensible.
Beyond the technological capabilities of RAMP is the important question of who. Who should have access to and own these tools? Who is building them and tailoring them for specific country contexts? Who is deploying these tools? Who is making decisions based on this data? Whose lives are affected by this technology? RAMP, a DevGlobal initiative, was designed and developed in concert with in-country decision makers and end users, giving small local governments and health ministries with limited resources a seat at the table.
As a species, we have faced societal and environmental disasters since the beginning of time. We will continue to face them until the end of time. By sharing innovative technologies openly and making them accessible to all, we can change the way that we respond to disasters and who has access to critical disaster relief.
For more information on RAMP, you can visit the project’s website at http://rampml.global/
DevGlobal Partners spans across Africa, India, and the United States supporting the world’s leading non-profits, companies, philanthropies, multilateral agencies, and government agencies on their innovation and sustainability initiatives. To learn more about how the team can facilitate event production and project management for your organization, contact info@dev.global.